La data science au service des administrations
8. Conclusion
Envie de lire plus de cas pratiques ? Consultez mon article sur l’analyse des sentiments (via Google Cloud).
8.1. Un retard d’adaptation
Les tentatives d’intégration de nouveaux modèles statistiques et de leurs applications informatiques dans le pilotage de projets, de programmes ou de politiques publiques ouvrent des voies prometteuses pour l’évaluation. La croissance exponentielle des données ainsi que la diffusion gratuite d’outils de développement accélèrent la recherche et les innovations dans le domaine. Si les organisations à but lucratif sont incitées à s’adapter, les acteurs associatifs et étatiques accusent un temps de retard et les évaluateurs peinent à innover dans leurs méthodes d’analyse.
8.2. Une évolution sans révolution
D’un autre côté, les cas abordés dans cette série d’articles tempèrent les fantasmes d’une évaluation automatisée : évaluer ne se résume pas à prédire des résultats et les problèmes d’efficacité ne se résolvent pas à coups d’optimisation d’AUC. La mission de l’évaluateur, autant pour l’aide à la décision que pour la production de connaissances, comporte une part irréductible de décisions et d’interactions humaines. Malgré cela, intégrer ces nouveaux outils demeure une opportunité irréfutable, tant pour la qualité des analyses que pour le développement des bonnes pratiques en évaluation.
« As Pete York at CommunityScience.com observes: “Herein lies the opportunity – we evaluators can’t battle the wave of big data and data science that will transform the way we do research. However, we can force it to have to succumb to the rules of objective rigor via the scientific method. Evaluators/researchers train people how to do it, they can train machines. We are already doing so.” (Personal communication 8/7/17) »
(Bamberger 2017b)
8.3. Ouvrir la réflexion sur les enjeux éthiques
La question éthique n’a été soulevée ici qu’à la marge, alors qu’elle reste fondamentale pour orienter le développement de nouveaux standards d’évaluation. Les enjeux soulevés par les nouvelles technologies appellent de nouvelles réflexions et il est urgent, pour les évaluateurs, de confronter les décideurs à leur existence.
Quelques enjeux éthiques soulevés par les NTICS
- La protection de la vie privée – dans un contexte de récolte massive et systématique d’informations, l’utilisateur peut être inconscient des données qu’il livre à des tiers (UN Global Pulse 2012, 24).
- La transparence des algorithmes d’intelligence artificielle – l’appropriation des données et du code source empêche la vérification et la régulation des technologies d’aide à la décision (Confédération suisse 2018, 31).
- L’utilisation des données – lorsque des sociétés tierces monopolisent le profit des informations tirées de publics vulnérables (Bamberger 2017b).
- La tentation de substituer les données traditionnelles par le big data – les données générées automatiquement peuvent représenter un gain d’efficience considérable pour les financeurs, qui peuvent choisir d’abandonner d’autres formes de récoltes de données et diminuer les interactions avec les bénéficiaires (Bamberger 2017c).
La relation que développeront les évaluateurs avec les NTICs demeure incertaine et le futur séparera les pionniers des suiveurs dans le développement de nouveaux standards de qualité. La révolution numérique marque le début d’une discussion excitante sur les promesses, les défis, les réussites et les échecs de la data science en évaluation. En prédire l’issue reste, ironiquement, hors de portée autant des humains que des machines.
À propos de Michael
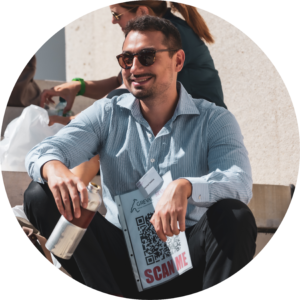
Consultant
Data Science | Evaluation | Web Dev
Je travaille comme entrepreneur et comme conseiller en organisation depuis 2016. Je suis spécialisé en data science, en évaluation et en développement d’applications web. J’ai aidé plusieurs organisations à moderniser leurs processus de travail (notamment @
) et je cultive une passion sans borne pour ce que le numérique peut apporter à la réalisation de projets. À côté, je dirige Sympa Bonnard, un e-commerce qui promeut une consommation éthique et locale. Je trouve mon épanouissement en soutenant des organisations à développer et à améliorer leurs projets, tout en leur transmettant les outils pour développer leur propre autonomie.
LinkedIn |
YouTube |
Écrivez-moi
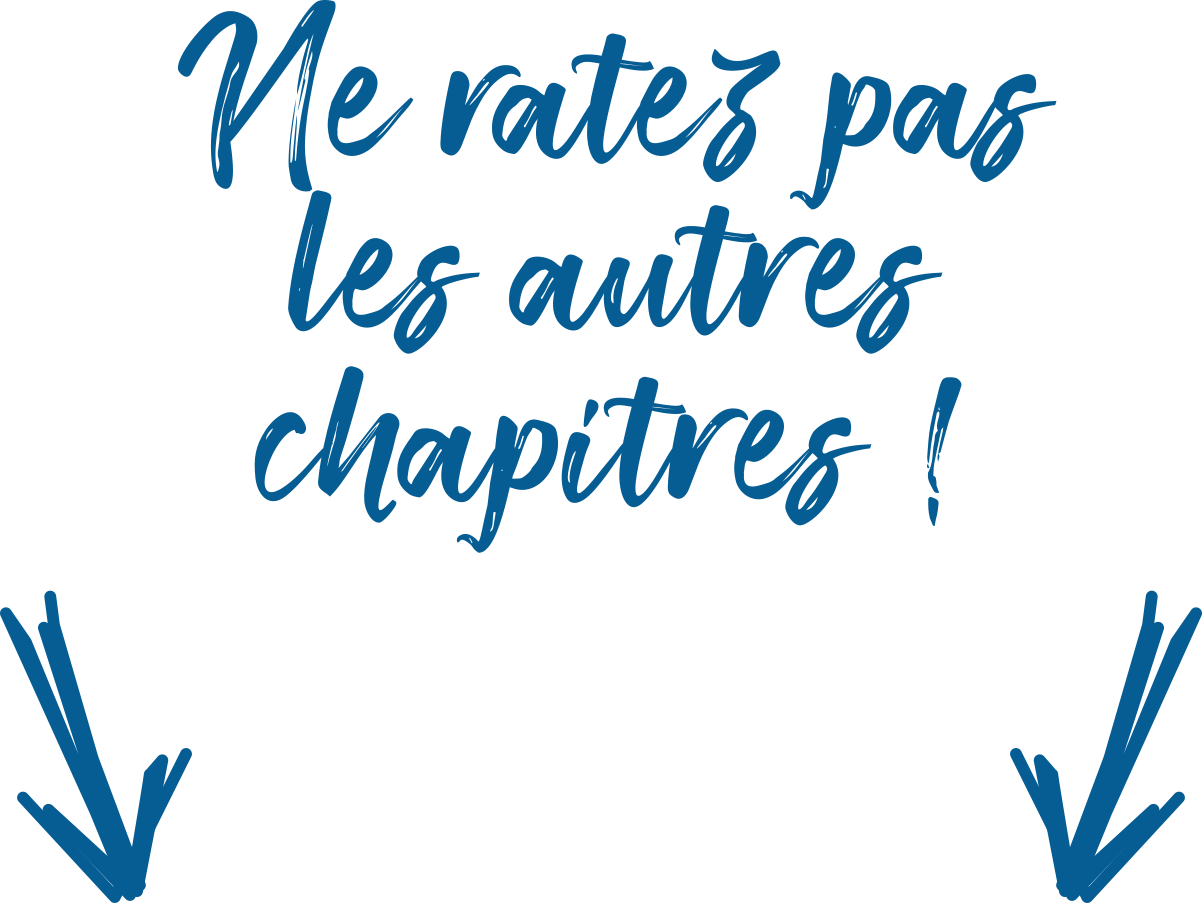
Les chapitres de ce travail
- Introduction : l’avènement de la data science grâce à la révolution numérique
- Survol de la discussion scientifique
- Quelques définitions
- Prédire les contraventions impayées de la ville Détroit
- La prédiction des pannes (ou maintenance prédictive)
- La gestion des tickets d’incident
- La détection de prestations erronées
- Conclusion
- Bibliographie
9. Bibliographie
- Alkin, Marvin C. Evaluation Roots: Tracing Theorists’ Views and Influences. Thousand Oaks: SAGE, 2004.
- Bamberger, Michael. Building bridges between evaluators and big data analysts. 05 09 2017b. http://merltech.org/building-bridges-between-evaluators-and-big-data-analysts/ (accès le 10 01, 2020).
- —. Commentaire en réponse à Satlaj Dighe. 17 09 2017c. http://merltech.org/the-future-of-development-evaluation-in-the-age-of-big-data/#comment-6 (accès le 10 01, 2020).
- —. The future of development evaluation in the age of big data. 19 08 2017a. http://merltech.org/the-future-of-development-evaluation-in-the-age-of-big-data/ (accès le 09 16, 2020).
- Berhane, Fisseha. Operations on word vectors. 2015. https://datascience-enthusiast.com/DL/Operations_on_word_vectors.html (accès le 09 27, 2020).
- Breiman, Leo. «Random forests.» Édité par Springer. Machine learning 45, n° 1 (2001): 5-32.
- Brownlee, Jason. airline-passengers.csv. 13 03 2018. https://github.com/jbrownlee/Datasets/blob/master/airline-passengers.csv (accès le 09 15, 2020).
- —. Discover Feature Engineering, How to Engineer Features and How to Get Good at It. 14 09 2014. https://machinelearningmastery.com/discover-feature-engineering-how-to-engineer-features-and-how-to-get-good-at-it/ (accès le 09 25, 2020).
- Campbell, Donald T. «Reform as Experiments.» American Psychologist 24, n° 4 (1969): 409–29.
- CC CDQ. Why Use Machine Learning to Improve Data Quality? 2018. 26 (accès le 2020 09, 2020).
- CES. What is Evaluation? 01 09 2016. https://evaluationcanada.ca/what-is-evaluation (accès le 09 19, 2020).
- Chen, Tianqi, et Carlos Guestrin. «XGBoost: A Scalable Tree Boosting System.» Arxiv, 08 2016: 785-794.
- Chen, Wo-Ruo, Yong-Huan Yun, Ming Wen, Hong-Mei Lu, Zhi-Min Zhang, et Yi-Zeng Liang. «Representative subset selection and outlier detection via isolation forest.» Anal. Methods 8, n° 39 (2016): 7225-7231.
- Christensson, Per. Data Science Definition. Sharpened Productions. 17 08 2017. https://techterms.com/definition/data_science (accès le 09 15, 2020).
- City of Detroit. Blight Violations Data. 01 10 2020. https://data.detroitmi.gov/datasets/blight-violations/data (accès le 10 01, 2020).
- CNRTL. ÉVALUATION, subst. fém. 2012. https://www.cnrtl.fr/definition/evaluation (accès le 09 19, 2020).
- Confédération suisse. «Stratégie Suisse numérique.» OFCOM. 09 2018. https://www.bakom.admin.ch/bakom/fr/page-daccueil/suisse-numerique-et-internet/strategie-suisse-numerique.html (accès le 10 01, 2020).
- Conway, Drew. The Data Science Venn Diagram. 26 03 2013. http://drewconway.com/zia/2013/3/26/the-data-science-venn-diagram (accès le 09 20, 2020).
- Filion, Adam. Predictive Maintenance with MATLAB A Prognostics Case Study. 2016. https://www.youtube.com/watch?v=9QUM5jtB0t4 (accès le 09 22, 2020).
- Finucane, Mariel McKenzie. «What Works for Whom? A Bayesian Approach to Channeling Big Data Streams for Public Program Evaluation.» merican Journal of Evaluation 39, n° 1 (03 2018): 109–22.
- Foote, Keith D. A Brief History of Machine Learning. 26 03 2020. https://www.dataversity.net/a-brief-history-of-machine-learning/ (accès le 09 15, 2020).
- Gartner. Gartner Reveals Five Major Trends Shaping the Evolution of Analytics and Business Intelligence. 02 10 2019. https://www.gartner.com/en/newsroom/press-releases/2019-10-02-gartner-reveals-five-major-trends-shaping-the-evoluti (accès le 09 16, 2020).
- Gavrilova, Yulia. Best Machine Learning Applications in 2020. 23 07 2020. https://serokell.io/blog/best-machine-learning-applications (accès le 09 15, 2020).
- Grimmer, Justin. «We Are All Social Scientists Now: How Big Data, Machine Learning, and Causal Inference Work Together.» PS: Political Science & Politics, 2015: 80–83.
- Higdon, Grace. Big Data to Data Science: Moving from ‘What’ to ‘How’ in MERL. 17 07 2020. http://merltech.org/wp-content/uploads/2020/07/3_MERL_Tech-Big-Data_FINAL_7.16.2020.pdf (accès le 10 01, 2020).
- IICD. «The Advantages of Digital Monitoring and Evaluation Solutions.» International Institute for Communication and Development. 06 2014. https://iicd.org/documents/the-advantages-of-digital-monitoring-and-evaluation-solutions/ (accès le 09 15, 2020).
- Jackson, Sally. Big Data Monitoring and Evaluation : A theoretical framework, tools, and lessons learned from practice. Draft v2.0, Jakarta,: United Nations Global Pulse, 2015.
- Kaggle. Detroit Blight Ticket Compliance: Help end blight in Detroit. 15 02 2019. https://www.kaggle.com/c/detroit-blight-ticket-compliance/rules (accès le 09 18, 2020).
- Knaus, Michael C. Double Machine Learning Based Program Evaluation under Unconfoundedness. Discussion Paper, St. Gallen: Institute of Labor Economics, 2020.
- Liu, Fei Tony, Kai Ming Ting, et Zhi-Hua Zhou. «Isolation Forest.» Proceedings of the 2008 Eighth IEEE International Conference on Data Mining. USA: IEEE Computer Society, 2008. 413–422.
- Macfarlan, Alice. Big Data and Evaluation – Use and Implications. 12 05 2015. https://www.betterevaluation.org/en/blog/big_data_in_evaluation (accès le 09 16, 2020).
- Madaus, George F., Daniel Stufflebeam, et Michael S. Scriven. «Program Evaluation.» Édité par Springer. Evaluation Models. Evaluation in Education and Human Services 6 (1983): 3-22.
- Math Vault. The Definitive Glossary of Higher Mathematical Jargon, Algorithm. s.d. https://mathvault.ca/math-glossary/#algo (accès le 09 15, 2020).
- Mayo, Matthew. Data Science Primer: Basic Concepts for Beginners. 08 2017. https://www.kdnuggets.com/2017/08/data-science-primer-basic-concepts-for-beginners.html (accès le 09 20, 2020).
- Mikolov, Tomas, Kai Chen, Greg Chen, et Jeffrey Dean. «Efficient Estimation of Word Representations in Vector Space.» Arxiv, 2013: 1-12.
- Mitchell, Tom M. «Key Ideas in Machine Learning.» Carnegie Mellon University. 04 12 2017. http://www.cs.cmu.edu/~tom/mlbook/keyIdeas.pdf (accès le 09 19, 2020).
- Mukarji, Olivier. Aid Agencies’ Use of Big Data in Human-centred Design for Monitoring and Evaluation. Geneva: Geneva Center for Security Policy, 2016.
- Nam, Ethan. Understanding the Levenshtein Distance Equation for Beginners. 26 02 2019. https://medium.com/@ethannam/understanding-the-levenshtein-distance-equation-for-beginners-c4285a5604f0 (accès le 09 26, 2020).
- Narkhede, Sarang. Understanding AUC – ROC Curve. 26 06 2018. https://towardsdatascience.com/understanding-auc-roc-curve-68b2303cc9c5 (accès le 09 19, 2020).
- NASA. Turbofan Engine Degradation Simulation Data Set. 2008. https://ti.arc.nasa.gov/tech/dash/groups/pcoe/prognostic-data-repository/ (accès le 09 22, 2020).
- Netten, Niels. «Exploiting Big Data for Evaluation Studies. In Proceedings of the 10th International Conference on Theory and Practice of Electronic Governance (ICEGOV ’17).» Association for Computing Machinery, 2017: 228–231.
- Office québécois de la langue française. Mégadonnées. 2020. http://gdt.oqlf.gouv.qc.ca/ficheOqlf.aspx?Id_Fiche=26507313 (accès le 09 20, 2020).
- Patton, Michael Quinn. Utilization-Focused Evaluation. Thousand Oaks: SAGE Publications, 2008.
- Pedregosa, et al. «Scikit-learn: Machine Learning in Python.» Journal of Machine Learning Research 12 (2011): 2825–2830.
- Petersson, Gustav Jakob. Cyber Society, Big Data, and Evaluation. New-York: AldineTransaction, 2017.
- Provost, Foster. «Data science and its relationship to big data and data-driven decision making.» BIG DATA 1, n° 1 (02 2013): 51-59.
- Quora. Quora Question Pairs: Can you identify question pairs that have the same intent? 16 03 2017. https://www.kaggle.com/c/quora-question-pairs/overview (accès le 09 27, 2020).
- Raftree, Linda, et Michael Bamberger. Emerging Opportunities: Monitoring and Evaluation in a Tech-Enabled World. Discussion paper, The Rockefeller Foundation, 2014.
- RDC. «Shooting your harddrive into space and other ways to practice responsible development data.» Responsible Data. 10 2014. https://responsibledata.io/wp-content/uploads/2014/10/responsible-development-data-book.pdf (accès le 09 20, 2020).
- République française. «Vocabulaire de l’intelligence artificielle.» Légifrance. 9 12 2018. https://www.legifrance.gouv.fr/jorf/id/JORFTEXT000037783813 (accès le 09 20, 2020).
- Schwab, Klaus. La Quatrième Révolution industrielle. Malakoff: Duno, 2017.
- Scriven, Michael. Evaluation Thesaurus. London: Newbury Park, 1991.
- SEFRI. Défis de l’intelligence artificielle. Rapport du groupe de travail interdépartemental Intelligence artificielle au Conseil fédéral. Rapport de groupe de travail, Bern: Confédération Suisse, 2019.
- Shalev-Shwartz, Shai, et Shai Ben-David. Understanding Machine Learning: From Theory to Algorithms. New York: Cambridge University Press, 2014.
- Taylor, Nick Paul. FDA approves diabetic retinopathy-detecting AI algorithm. 13 04 2018. https://www.fiercebiotech.com/medtech/fda-approves-diabetic-retinopathy-detecting-ai-algorithm (accès le 09 2020, 15).
- Tipping, Michael E., et Christopher M. Bishop. «Probabilistic Principal Component Analysis.» Journal of the Royal Statistical Society: Series B (Statistical Methodology) 61, n° 3 (06 1999): 611-622.
- UN Global Pulse. «Big Data for Development: Challenges and Opportunities.» UN Global Pulse. 05 2012. https://www.unglobalpulse.org/document/big-data-for-development-opportunities-and-challenges-white-paper/ (accès le 09 15, 2020).
- UN Global Pulse. Integrating Big Data into the Monitoring and Evaluation of Development Programmes. Tamara Karaica and Felicia Vacarelu, 2016.
- UNDP. «Innovations in Monitoring & Evaluation.» 3rd International Conference on National Evaluation Capacities. São Paulo, 2013. 25.